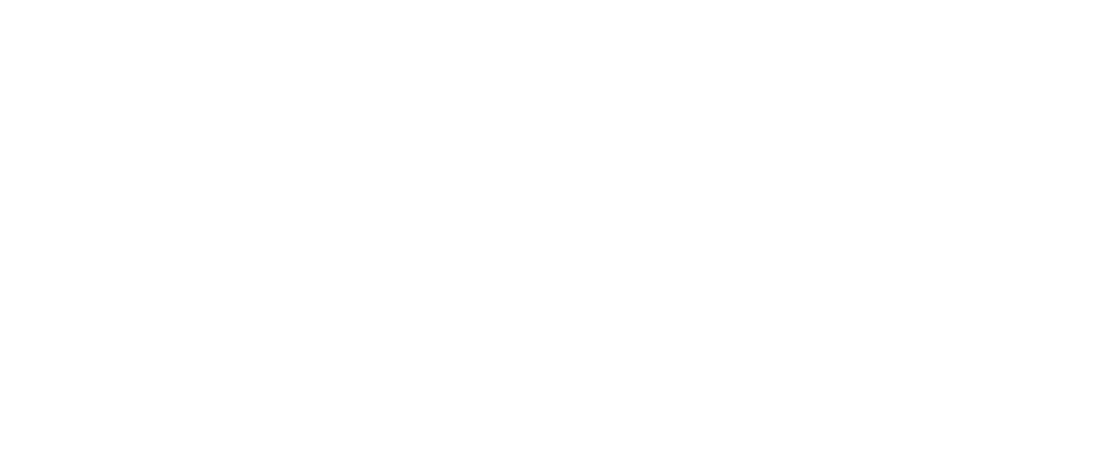
AI for Science
AI for Science is a programme focused on accelerating the AI capabilities of large-scale
experimental facilities funded and managed by STFC.
It covers five key areas:
- AI for Data Analysis: Equipping the scientific community with state-of-the-art data analysis techniques to maximize insights from large-scale experimental datasets produced by STFC facilities. Our tools and methodologies are designed to handle complex data challenges and drive ground breaking discoveries.
- AI for Science Benchmarks: Creating and refining benchmarks to evaluate the performance and effectiveness of various AI models across different systems in scientific applications. Our objective is to promote openness and advance scientific research by providing transparent and rigorous evaluation metrics.
- AI for Scientific Image Processing: Applying advanced AI techniques to enhance image quality and identify objects within scientific images.
- AI for Smart Facilities: Developing cutting-edge instrumentation and control algorithms to ensure STFC facilities are advanced, automated, and intelligent.
- Core AI Research: Addressing fundamental challenges in AI that impact STFC-funded facilities and programs.
5 Key Areas in Detail
AI for Data Analysis
Focuses on developing and applying advanced machine learning and AI techniques to analyse the large experimental datasets generated by STFC facilities. Working closely with facility scientists, the group gains a deep understanding of the unique characteristics of different data types, enabling tailored techniques for each dataset. By leveraging these techniques, they enhance data interpretation, uncovering patterns and relationships that would be difficult to detect using traditional methods.
1. Advanced Representation Learning for 4D-STEM Data Interpretation
2. Enhancing the Interpretability of X-ray Photon Correlation Spectroscopy through Representation Learning
3. Free-Form Inversion for Small-Angle Scattering (SAS)
4. Learning Pose Variability in Cryo-EM Data through Representation Learning
5. Pulse Shape Discrimination and Saturated Pulse Reconstruction Using Kalman Filtering
6. Unveiling Crystal Growth Patterns through Advanced Representation Learning
7. Unveiling Galaxy Structures through Representation Learning in Galaxy Zoo Data
AI for Science Benchmarks
Focuses on testing various AI model architectures across different high-performance computing systems to evaluate the performance, scalability, and efficiency of these models in real-world scientific applications. By rigorously benchmarking models on different hardware setups, the theme provides valuable insights into which architectures perform best under specific conditions, promoting transparency and enabling the scientific community to choose the most suitable models and systems for their research.
1. The SciML Benchmark Suite
AI for Scientific Image Processing
Dedicated to advancing techniques that enhance the quality of complex scientific images and improve object detection within them. By collaborating closely with researchers, the group develops customized algorithms for tasks like noise reduction, image segmentation, object recognition, and anomaly detection. These methods are applied across a wide range of images generated by STFC facilities, enabling scientists to extract precise information, visualize microscopic structures more effectively, and automate the analysis of intricate datasets that would otherwise require manual effort.
1. Accelerating Inelastic Neutron Scattering Simulations with Generative Machine Learning
2. Alignment of Frames from Stochastic Optical Reconstruction Microscopy (STORM)
3. Automated Annotation and Analysis of 3D Cellular cryoFIBSEM Imaging
4. Automated Detection of Objects of Interest in Fluorescence Localisation Imaging with Photobleaching
5. Cloud Segmentation in Sentinel-3 SLSTR Satellite Images
6. Detecting Bragg Peaks in 3D X-ray/Neutron Images
7. Filtering Contaminants in Picked CryoEM Particles
8. Machine Learning for Classifying Inelastic Neutron Scattering
9. SMART-EM: Automating Cryo-EM Particle Extraction
10. Supervised Segmentation for X-ray Tomography on Prehistoric Fish Data
AI for Smart Facilities
Dedicated to developing intelligent systems that automate and enhance the operation of STFC facilities. By collaborating closely with engineers and scientists, the theme designs solutions for automated calibration, diagnostics, and facility management for ISIS, Diamond, CLF and UKATC. Through the application of smart technologies, the theme aims to improve operational efficiency, minimize downtime, and enable next-generation capabilities, ensuring that STFC facilities continue to lead in innovation.
1. Auto-calibration of Scanning Transmission Electron Microscopes using Reinforcement Learning
2. Development of a Virtual Diagnostic for Estimating Key Beam Descriptors
3. FAIR-MAST: A Fusion Device Data Management System
4. FLIMP-autofocusing: Autofocusing for Optimal Image Quality in Fluorescence Localisation Imaging with Photobleaching
5. Laser Induced Damage Prediction in Real-time
6. Machine Learning Electron Probes for Ptychographic Reconstruction
7. Machine Learning for Adaptive Optics and Hyperspectral Imaging via Synchrotron InfraRed
8. Realtime Reduction, Monitoring and Early Stopping for ISIS-SANS
9. Reinforcement Learning-Driven Autofocusing for Fluorescence Localization Imaging with Photobleaching
10. Using Surrogate Models to Assist Accelerator Tuning at ISIS
Core AI Research
The theme is not only focused on developing machine learning technologies for scientific data but is also committed to making innovative contributions to the field of machine learning. Our members are engaged in various topics, including representation learning, physics-informed neural networks, graph neural networks, 3D image segmentation, AI benchmarking, large language models, and related teaching and tutoring.
1. Active Learning Materials Properties with Graph Neural Nets
2. Fully Unsupervised 2D/3D Image Segmentation
3. Global Optimality for Non-linear Constrained Restoration Problems via Invexity
4. Global Convergence of Alternating Direction Method of Multipliers for Invex Objective Losses
5. Orthogonality-Enforced Latent Space in Autoencoders: An Approach to Learning Disentangled Representations
6. Padding-Free Convolution Based on Preservation of Differential Characteristics of Kernels
7. The Federation Project: Machine Learning for Science
8. Zero coordinate shift: Whetted automatic differentiation for physics-informed operator learning
Projects
National Platform for Research Technical Professionals on AI for Science and Engineering (NPRAISE)
Read more about NPRAISE here.
AI for Realistic Science (AIRS)
For more information and all enquiries, please contact us.
Blueprinting AI for Science at Exascale (BASE-II)
For more information, visit the BASE-II website.
SciMLBench
To explore SciMLBench, please click here.